TH: “Like” 讚 is a short story collected in Mary Wong’s Surviving Central 中環人, which won the “25th Secondary School Students’ Best Ten Books Award.”

Chandler strolled into the sleek modern Haneda Airport, wearing a casual ensemble. He handed over his passport and air tickets to the counter clerk, and the check-in process proceeded swiftly, devoid of the usual crowds and hassle. With his boarding pass in hand, he made his way towards the lounge designated for business class passengers, sporting a stylish Comme des Garçons T-shirt. Even on a typically damp and chilly day in Tokyo, he felt a distinct sense of contentment.
“Have a pleasant journey!” the clerk chimed in politely, her eyes drawn to the colourful checked pattern of his T-shirt.
The view beyond the oversized glass windows seemed more vivid than any scene on a movie screen. Chandler had just sealed his first business deal at the age of twenty-eight. As he eased into a comfortable chair, he gazed out at the runway, savouring the moment. The lounge exuded an air of tranquillity, occasionally disrupted by the muffled laughter emanating from the television, only to be quickly absorbed by the serene ambiance. Though Chandler spoke Japanese fluently, he remained a bit distant from Japanese culture. Yet, at this particular moment, he had no desire to delve into the reasons behind the airport’s pervasive hush. He simply relished the sight of planes ascending and descending, lost in the visual serenity that the absence of noise provided.
Deep down, Chandler was aware that his command of the Japanese language wasn’t truly proficient, and he certainly wasn’t a qualified interpreter. His journey into interpretation had been triggered by his uncle’s misapprehension and his family’s expectation that he could earn a living at it. Accompanying his uncle to Tokyo to negotiate a business deal with a fashion company, Chandler found himself acting as interpreter for half a day, drawing upon all the knowledge of the Japanese language he had amassed during three years of college. He gave it his all, deriving contentment from his performance that would sustain him for an entire year without work. With over an hour remaining until boarding, Chandler eventually grew weary of the captivating airport views. He sipped his beer as he reached for his laptop, intending to peruse his Facebook feed. After a few days away from Hong Kong, nothing seemed to have changed, but he diligently clicked the “like” button for various posts as per his usual routine. He was neither opinionated nor inspirational; simply “liking” was the extent of his online engagement.
With ample time still on his hands, Chandler turned his attention to his somewhat neglected email account. After a couple of beers, he had nearly forgotten about his passport—a detail he quickly remembered and secured. However, his inbox was littered with emails he had grown to detest, most of them promotional. Despite his reservations, he opened them one by one in the faint hope of finding something remotely interesting. After a few minutes, his curiosity was piqued by the realisation that someone had been sending him an email every day for the past three days. The subjects of these emails were identical: “STEALING”. Intrigued, he decided to open the first one, and it left him stunned. Chandler rarely encountered such lengthy emails composed in Chinese. The sender was someone named Chuck Shum.
Chuck and Chandler had been students at the same college, though they never shared a classroom. Their only connection was their mutual preference for the same restroom on a specific floor of one campus building. They had bumped into each other a few times, engaging in casual conversations to alleviate the inherent awkwardness of these encounters. They had exchanged a few emails about concerts, but their interactions were limited. Chuck and Chandler were acquaintances who only ever conversed when they found themselves in the restroom simultaneously. Chandler’s mind was clouded by alcohol, which had a tendency to make his thoughts wander. He enjoyed these mental drifts and experienced them quite frequently. It felt like reconnecting with an old friend.
“Ah, Chuck Shum! The guy from the third-floor restroom!” Chandler exclaimed with a sense of familiarity. He ordered a coffee to clear his mind, and his attention shifted back to the laptop. It was as if he had stumbled upon a new, captivating toy. In the midst of the otherwise dull lounge, he had finally found something intriguing. The first “STEALING” email read:
Dear Chandler,
Do you remember me from our chance encounters in the third-floor restroom five years ago? It’s been quite a while since we last crossed paths at that music concert. How have things been for you? I’m reaching out to share an unsettling experience—someone has stolen two hundred dollars from me. I find myself wondering, why me? Why would someone target me in this way? What could be the reason behind it? For years now, I’ve been diligently working as a teacher at a rather challenging high school. I’ve put in more effort than most of my colleagues here. Just yesterday, something happened that left me perturbed. Miss Lin, who sits behind me, decided to put on a performance for our programme director. In the process, her paperwork ended up scattered across my desk. Instead of making a fuss, I took it upon myself to clean up the mess and complete the tasks at hand. You see, I’ve always been this way—I’ve never scolded my students and have always protected them. Even when they were caught up in plagiarism incidents, I opted to give them opportunities to make amends. And yet, these ungrateful youngsters seem to admire our rather uncouth physical education teacher above anyone else. They describe him as “hot” and “cool”. I can’t help but wonder, what have I done wrong? Why can’t they simply leave me be? Why would someone resort to stealing from me? I’m genuinely frightened, Chandler—the fear is real. Have I unintentionally offended someone? Are they seeking revenge?
Chuck Shum
Chandler finished reading the email. The airport lounge remained eerily quiet, now populated with more middle-aged men in suits. His heart began to race, its rhythm out of sync with the serene atmosphere around him. Why was Chuck so agitated over two hundred dollars? If he was unhappy at work, he could simply take a break, perhaps go skiing in Switzerland. Chandler had taken up skiing in recent years and often visited European slopes with friends he’d met online. These were acquaintances he only encountered in the snowy mountains. Chuck could join him in Switzerland, leaving his school troubles behind. It seemed so straightforward. Chandler didn’t respond immediately but continued reading.
Hi Chandler,
Someone has taken another hundred dollars from me! Why is this happening only to me? Let me fill you in on who I think this thief might be. I had lunch with Mr Chan, who works in the same programme, to gather more insights about the school. During our conversation, I vented about our programme director, knowing that they had a strained relationship. Mr Chan responded with great enthusiasm and talked non-stop throughout our meal. After we returned to our office, I went to the restroom to brush my teeth. While I was there, I overheard two individuals conversing right outside the door. I stealthily approached the door and pressed my ear against it. I am almost certain it was Mr Chan and the program director. There’s no doubt in my mind. I didn’t dare to open the door, so I waited for their voices to fade. The experience sent shivers down my spine. When I returned to the sink and continued brushing my teeth, I brushed until my gums bled, serving as a painful reminder that it was time for class. That’s when it hit me. They were plotting against me, driving me to paranoia and desperation…
Chuck
Chandler began to question why Chuck had reached out to him about all this. They had never exchanged phone numbers, and even if they were to stand face to face at that moment, they might not recognise each other. Nonetheless, this email had stirred something within Chandler. He sensed there was more to the story than met the eye. He usually steered clear of complicated matters, but Chuck’s predicament piqued his curiosity. Who was behind the theft of Chuck’s money? As his anxiety grew, he opened the third email.
I’m delighted to inform you, Chandler, that another theft has occurred at the school. This time, I wasn’t the victim. Now I can sit back and focus on grading.
Chandler felt utterly disappointed. Was that all there was to it? Where were the complications he had anticipated? He headed for the cabinet and retrieved a bottle of local sake with a pronounced hint of bitterness. A few shots might be just what he needed to alleviate the tedium of his surroundings. Returning to the window, he attempted to while away the remaining half-hour by watching planes take off. Yet his thoughts kept returning to the image of bloodied gums dripping into the basin from Chuck’s mouth. He felt an odd sense of excitement amid the calmness and pushed back his screeching chair. Returning to his laptop, he clicked “Reply” and typed the word he knew best:
“Like.”
How to cite: Song, Chris and Mary Wong. “Like.” Cha: An Asian Literary Journal, 11 Sept. 2023, chajournal.blog/2023/09/11/like.


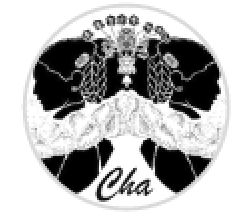
Mary Shuk-Han Wong 黃淑嫻 (author) is a Hong Kong writer. Her short story collection Surviving Central (中環人; 2013) received the “25th Secondary School Students’ Best Ten Books Award.” Her essay collections include How to Live the Sad Days (悲傷的日子如何過; 2021), Against the Grain (亂世破讀; 2017), and From Kafka (理性的遊藝:從卡夫卡談起; 2015). She has also published an online poetry collection, Cave Whispers (絕地抒情; 2022), in collaboration with Hong Kong composer and photographer. She was the co-producer and literary advisor of two literary documentaries: 1918: Liu Yichang (1918:劉以鬯紀錄片; 2015) and Boundary: Leung Ping Kwan (東西:也斯紀錄片; 2015).
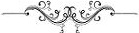
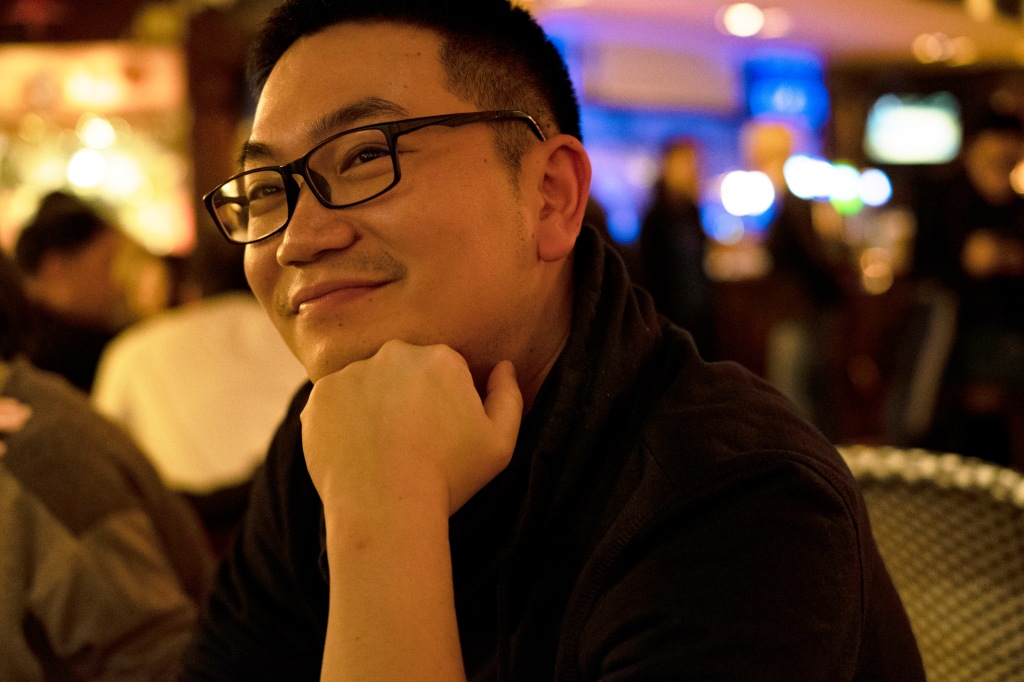
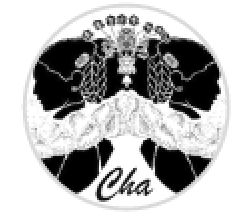
Chris Song (translator) is a poet, editor, and translator from Hong Kong, and is an assistant professor in English and Chinese translation at the University of Toronto Scarborough. He won the “Extraordinary Mention” of the 2013 Nosside International Poetry Prize in Italy and the Award for Young Artist (Literary Arts) of the 2017 Hong Kong Arts Development Awards. In 2019, he won the 5th Haizi Poetry Award. He is a founding councilor of the Hong Kong Poetry Festival Foundation, executive director of the International Poetry Nights in Hong Kong, and editor-in-chief of Voice & Verse Poetry Magazine. He also serves as an advisor to various literary organisations.
